2025 APAC Data & AI Trends: AI-Driven Business Transformation – Are You Ready for the Change?
Back to all articles
In this article, we explore the key trends shaping 2025 and business transformation. These trends collectively reflect the evolving landscape of technology, highlighting the need for businesses to adapt and innovate to stay competitive.
As we step into 2025, the APAC region stands at a critical point defined by rapid technological advancements and evolving consumer preferences. According to IBM's 'APAC AI Outlook 2025' report, APAC enterprises are moving beyond AI experimentation to maximize the impact of their AI investments. More than half (54%) now believe that AI will deliver long-term benefits in areas such as innovation and revenue generation.
Organizations in this diverse landscape face challenges such as intense competition and the need for agile responses to rapidly changing market demands. However, these hurdles also present unique growth opportunities. Companies that effectively harness data are not only navigating these complexities but also gaining a competitive edge.
In this article, we explore the key trends shaping 2025 and business transformation, which include understanding data-centric AI, AI’s return on investment, whether to buy or build AI tools, sustainability as a competitive advantage, and finally, Agentic AI. These trends collectively reflect the evolving landscape of technology, highlighting the need for businesses to adapt and innovate to stay competitive.
The Role of Data-Centric AI in Machine Learning
Data-centric AI emphasizes the importance of high-quality data as the foundation for effective AI systems. Rather than focusing solely on algorithm optimization, this approach prioritizes the curation, labeling, and structuring of data. According to O'Reilly, "48% of businesses use machine learning, data analysis, and AI tools to maintain data accuracy."
Key principles of this approach include comprehensive data collection, which involves acquiring extensive datasets from diverse sources, such as structured data, unstructured data, and real-time data streams. Equally important is ensuring data quality; this means maintaining clean, accurately labeled data that is representative of the relevant business problem and domain. To achieve high data quality, effective techniques such as data cleaning, preprocessing, and augmentation are essential, as they enhance the reliability and applicability of the data in machine learning applications. To illustrate a real-world application, consider Tesla, which has seen significant advancements in its autopilot and full self-driving capabilities coinciding with the rise of data-centric AI. Tesla’s technology relies heavily on the vast amounts of data collected from millions of miles driven by its users. This extensive dataset is utilized to train and refine Tesla’s AI algorithms, ultimately enhancing the performance and safety of their autonomous systems.
This shift towards data-centric AI underscores the evolving landscape of machine learning, which has traditionally relied on a model-centric approach, making models more accessible through platforms like GitHub. However, effective machine learning now demands accurately curated data alongside robust models. As today's models become more sophisticated, the greatest returns will stem from prioritizing data quality, with iterative development increasingly driven by data insights to determine success or failure.
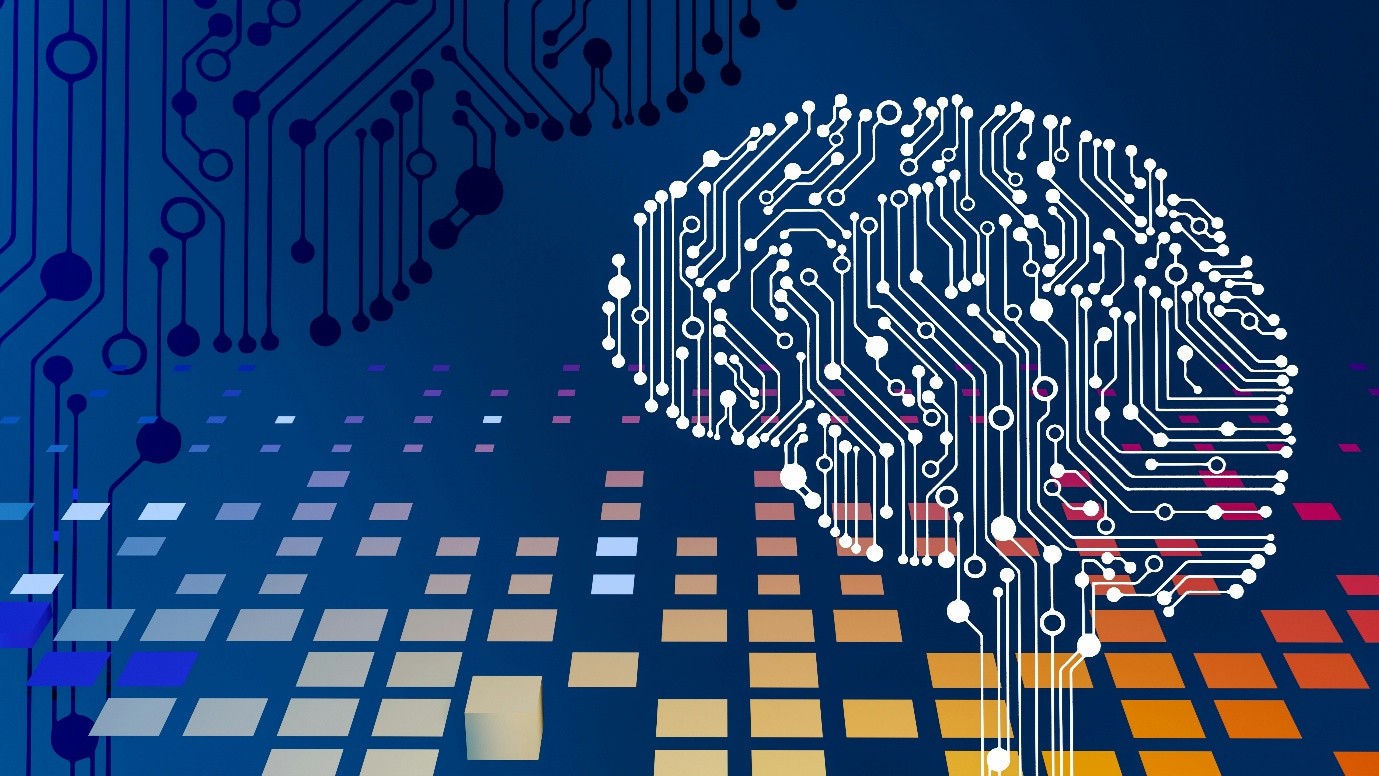
Understanding AI ROI
It’s crucial for companies to evaluate the return on investment (ROI) associated with their AI initiatives, yet many still overlook the importance of effective monitoring. Fortunately, this trend of hastily adopting AI without strategic oversight is diminishing. Organizations are now prioritizing the need to extract tangible, quantifiable value from their AI investments.
A recent survey from Google found that 60% of organizations have implemented GenAI in production, with 86% reporting revenue increases of 6% or more. This highlight shows companies who actively assess their AI investments are more likely to see tangible benefits and competitive advantages. By focusing on measurable outcomes, such as cost reductions, enhanced productivity, and revenue growth, organizations will maximize the value derived from their AI initiatives and ensure sustainable success.
The substantial ROI that manufacturers are achieving through the adoption of Generative AI (GenAI) underscores the necessity of measuring and understanding AI investments. For instance, companies like Siemens have reported significant cost savings by using AI for predictive maintenance, which helps reduce downtime and prolong equipment lifespan. By analyzing performance data and predicting failures before they occur, Siemens has improved operational efficiency and reduced maintenance costs by up to 30%. Similarly, in the automotive sector, companies like Ford are using GenAI to streamline quality assurance processes, resulting in faster time-to-market for new vehicles and increased overall productivity.
As Forrester notes, by 2025, businesses will increasingly emphasize the need to demonstrate ROI and concrete value from AI initiatives, ensuring their investments deliver real benefits rather than merely keeping up with industry trends.

Eki offer: AI Core Platform - Ekimetrics
Gen AI Tools: Buy or Build
As organizations navigate the integration of AI into their operations, a critical decision emerges: should they buy pre-built AI solutions or build custom systems? This choice, often framed as "buy vs. build," is shaping the future of AI deployment and has significant implications for business strategy.
The trend toward rapid deployment of enterprise-ready AI products underscores the urgency of this decision. Pre-built solutions offer the advantage of quick implementation, allowing businesses to integrate advanced AI capabilities without lengthy development cycles. With the emergence of tools like DeepSeek, organizations find that the cost of adopting pre-built solutions can be further reduced.
In navigating this buy vs. build dilemma, decision-makers should consider several factors:
• Business Objectives: Is AI a core component of your strategy, or is it a supporting tool? Core applications may warrant a custom build to ensure precise alignment with business goals.
• Time to Market: How urgently do you need to deploy an AI solution? If immediate implementation is crucial, off-the-shelf solutions can provide a faster path to integration.
• In-House Expertise: Assess your team's current AI capabilities. If your organization lacks the necessary skills for custom development, purchasing a pre-built solution may be more viable.
• Cost Considerations: While buying may appear more cost-effective initially, custom solutions can lead to lower total costs of ownership in the long run, particularly as business needs evolve.
• Scalability and Control: Custom-built solutions offer better control over data and processes, reducing fragmentation and enhancing the ability to adapt to future requirements.
Ultimately, the choice between buying and building AI solutions isn't binary; many organizations may find value in a hybrid approach, leveraging both pre-built products and custom features. By carefully evaluating the advantages of each option, businesses can make informed decisions that support their strategic objectives and drive successful AI adoption.

Eki offer: AI Core Platform - Ekimetrics
AI, Automation and ESG
In 2025, sustainability will evolve into a fundamental business strategy, significantly enhanced by advancements in AI. Companies that prioritize eco-friendly practices and leverage AI technologies can optimize resource use, reduce waste, and develop sustainable products or services more efficiently.
By integrating AI into their sustainability efforts, businesses can analyze vast amounts of data to identify areas for improvement and create innovative solutions that meet the growing consumer demand for environmentally responsible products. According to YouGov’s multi-market poll, more than three in five consumers (71%) globally consider social factors before making a purchasing decision, underscoring the importance of a commitment to positive social and environmental impact for brands.
Key Predictions for Advancing Sustainability and ESG Initiatives by 2025:
• Organizing and Governing ESG Data: By 2025, organizations will increasingly prioritize the organization and governance of ESG data from diverse sources, including internal processes and supply chains. Robust data governance frameworks will become essential for ensuring data reliability and compliance. As companies integrate ESG data with existing business intelligence systems, they will gain enhanced insights, while advanced analytics will enable the identification of trends, risks, and opportunities for improvement.
• Implementing Effective ESG Measurement: The use of AI in ESG measurement will become more prevalent, allowing organizations to tackle challenges in data utilization more effectively. AI-driven solutions will analyze a variety of data sources, including sensors, meters, GIS data, and hydraulic models, providing actionable insights related to environmental impacts, operational efficiency, and compliance metrics.
• Identifying and Selecting AI-Driven Use Cases: As sustainability efforts evolve, the selection of the right AI-driven use cases will be critical. Organizations will assess their operations to pinpoint areas where AI can deliver substantial benefits, such as energy optimization and waste reduction. Tailoring AI solutions to align with strategic objectives will ensure effective problem-solving.
For instance, Unilever has integrated AI into its sustainability initiatives by using data analytics to reduce water usage in production processes and optimize supply chain logistics. By analyzing vast amounts of data, Unilever identifies areas for improvement and develops innovative solutions that meet the growing consumer demand for environmentally responsible products. This dual focus not only strengthens brand image but also builds deeper connections with eco-conscious consumers, driving loyalty and competitive advantage in the marketplace.
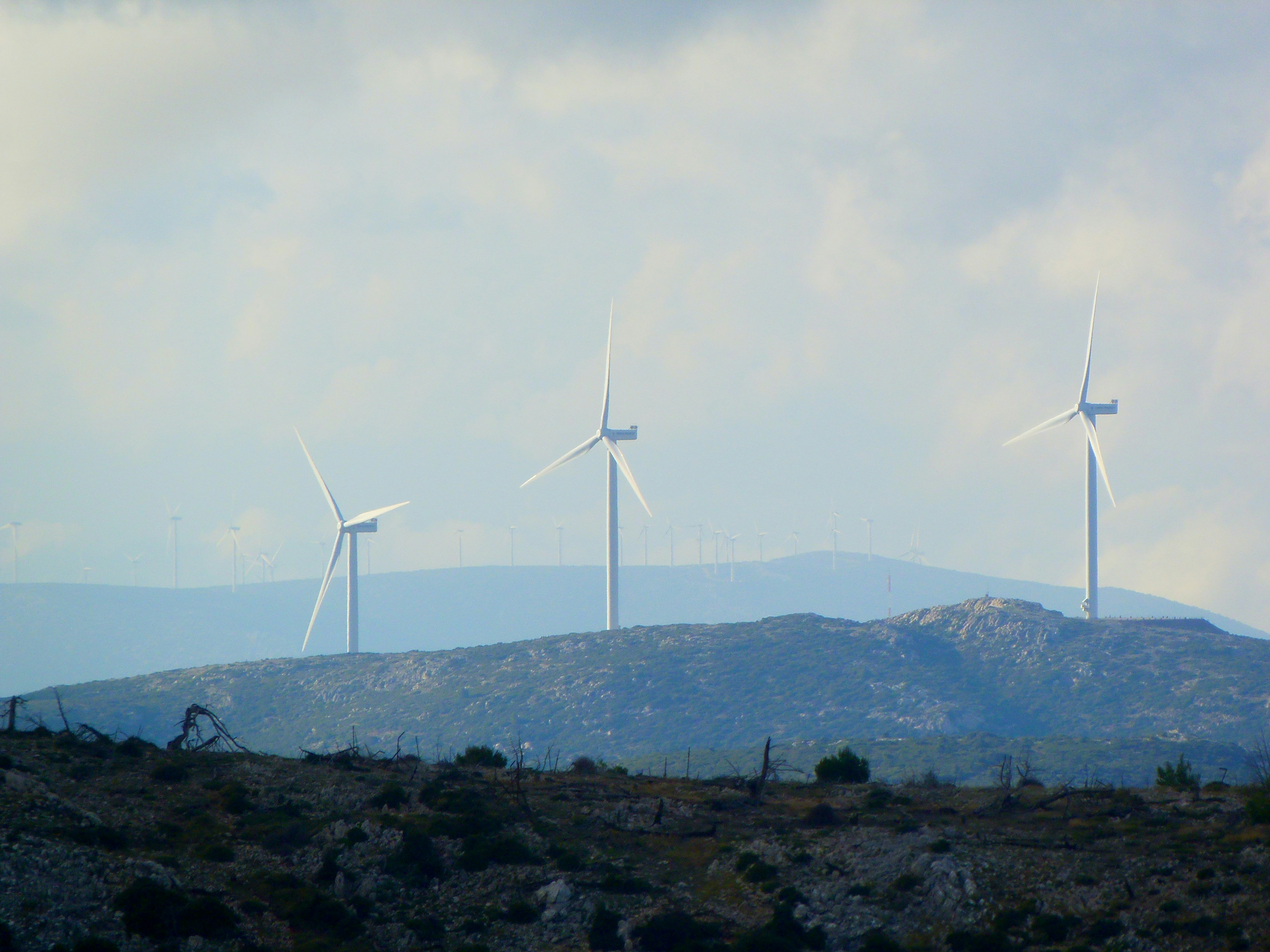
Eki offer :
Data Science Transformation to oversee an insurer’s ESG roadmap - Ekimetrics
AI For Sustainability - Ekimetrics
Agentic AI: A Game Changer for Business and Marketing
Agentic AI represents a significant evolution in artificial intelligence, characterized by systems that can autonomously perform tasks, make decisions, and learn from their environments without constant human intervention. Unlike traditional AI models that rely heavily on predefined algorithms and human oversight, Agentic AI systems possess the capability to adapt and optimize their actions based on real-time data and experiences. This autonomy positions Agentic AI as a powerful tool for organizations aiming to enhance operational efficiency and drive innovation.
The importance of Agentic AI is profound in today's competitive and dynamic landscape. By deploying these advanced systems, organizations can significantly reduce the time and resources required for decision-making processes. Agentic AI can analyze vast amounts of data, identify patterns, and generate insights at speeds far beyond human capabilities. This enables businesses to make informed strategic decisions swiftly, allowing them to respond effectively to market changes and emerging opportunities.
By 2025, Agentic AI is set to transform marketing in several key ways:
• Hyper-Personalization
Agentic AI analyzes extensive datasets to understand individual consumer behaviors, enabling marketers to deliver personalized content and offers. For example, AI agents can autonomously identify cross-sell and upsell opportunities based on customer profiles.
• Automated Content Creation
Agentic AI streamlines the production of high-quality content in various formats—text, images, and videos.
• Data-Driven Insights
Agentic AI systems analyze market trends and consumer behaviors to offer actionable insights, empowering marketers to make informed decisions. Companies like Omneky utilize AI for generating personalized ad content, enhancing campaign effectiveness.
• Optimized Campaign Management
AI agents autonomously plan, execute, and refine marketing campaigns across channels, adjusting strategies based on performance data and audience feedback. Platforms like Taboola are introducing AI assistants to help small and medium-sized businesses with ad campaign management.
.jpg)
Conclusion
As we enter 2025, the call to action for organizations in the APAC region is unmistakable: embracing Generative AI is not just a strategic choice but a necessity for thriving in a rapidly evolving landscape.
At Ekimetrics, we believe that adopting a strategic approach is crucial before committing to investments in Generative AI. Companies should first assess their current position and business priorities to ensure that any deployment aligns with their specific needs. While Generative AI has the potential to optimize operations, deploying it without a clear use case can lead to wasted resources. Understanding the potential business impact is crucial, as it helps maintain focus on the fundamental elements that drive success.
The journey ahead is not merely about keeping pace with change; it’s about leading the charge into a future where businesses in APAC are not just reactive but visionary, setting the standard for responsible growth and digital transformation across the region.
1. Google: https://cloud.google.com/transform/manufacturing-gen-ai-roi-report-dozen-reasons-ai-value
2. Business insider: https://www.businessinsider.com/ai-siemens-predict-industrial-maintenance-machine-infrastructure-equipment-costs-productivity-2024-11
3. Master Of Code: https://masterofcode.com/blog/generative-ai-in-manufacturing
4.YouGov: https://business.yougov.com/content/46185-global-market-analysis-esg-importance-consumer-purchase-decisions
5. Aim research: https://aimresearch.co/market-industry/how-unilever-is-leveraging-ai-to-drive-innovation-and-sustainability
6. O’Reilly: https://www.oreilly.com/radar/the-state-of-data-quality-in-2020/
7. Cloud Factory: https://cloudfactory.com/blog/three-waves-of-ml