The International Conference on Learning Representations (ICLR) 2024 workshop has accepted our scientific paper on ClimateQ&A
Back to articles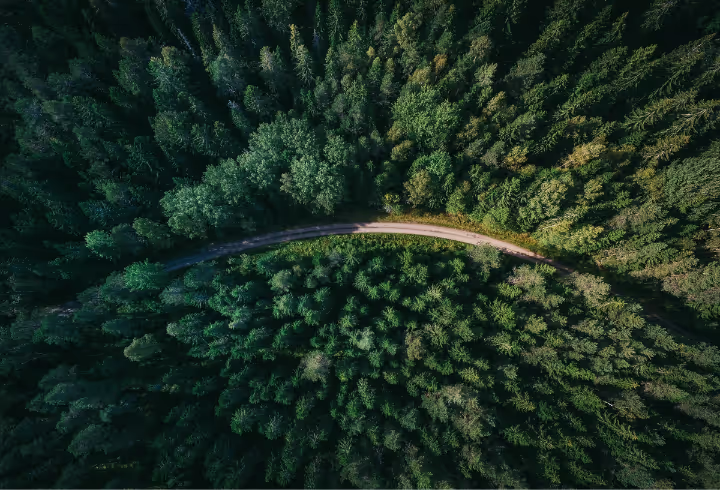
From May 7 to 11, Ekimetrics is in Vienna, Austria, for the ICLR 2024: Tackling Climate Change with Machine Learning.
From May 7 to 11, Ekimetrics is in Vienna, Austria, for the ICLR 2024: Tackling Climate Change with Machine Learning. We’ll present a poster of our scientific paper “ClimateQ&A: bridging the gap between climate scientists and the general public”, written by our team of experts led by our Responsible AI Lead, Annabelle Blangero.The ICLR is the premier gathering of professionals dedicated to advancing the branch of artificial intelligence called representation learning, generally known as deep learning. The Scientific Committee reviewed our paper and we’re pleased to tell you that it was the only paper accepted in the “Behavioral and Social Science | Climate Change AI” category.ClimateQ&A is a two-way tool that democratizes information and provides insights on climate change for the general publicOur research paper investigates public views on climate change and biodiversity loss by analyzing the questions posted on our ClimateQ&A platform. ClimateQ&A is a conversational agent that uses LLMs to respond to queries based on over 14,000 pages of scientific literature from IPCC and IPBES reports. It aims to make climate and biodiversity science more accessible to the public using generative AI. We named our paper “Bridging the gap” as it’s a two-way tool. Thanks to the conversational agent, the public addresses their concerns and questions to scientists who analyze their queries.We extracted a sample of 7,000 questions posted to the platform in April 2023, excluding repeat questions (exact matches) and unclassifiable questions (e.g., short follow-up questions by the user). This left a sample of 3,425 questions. We then conducted clustering based on dense embeddings of the questions (bge-base-en-v1.5). We automated initial cluster naming using BERTopic. Using this NLP approach, we obtained a categorization of questions across 130 different clusters representing salient topics. We manually reviewed each cluster to identify recurring categories and sub-categories. Finally, we annotated each cluster based on whether the user had expressed a general or personal inquiry.The International Conference on Learning Representations accepting our paper is a testament to our GenAI capacities and advanced knowledge in Natural Language Processing (NLP) clustering algorithms, combined in our innovative tool.Want to know more? Read our popular science article: Investigating public perceptions of climate change using ClimateQ&A