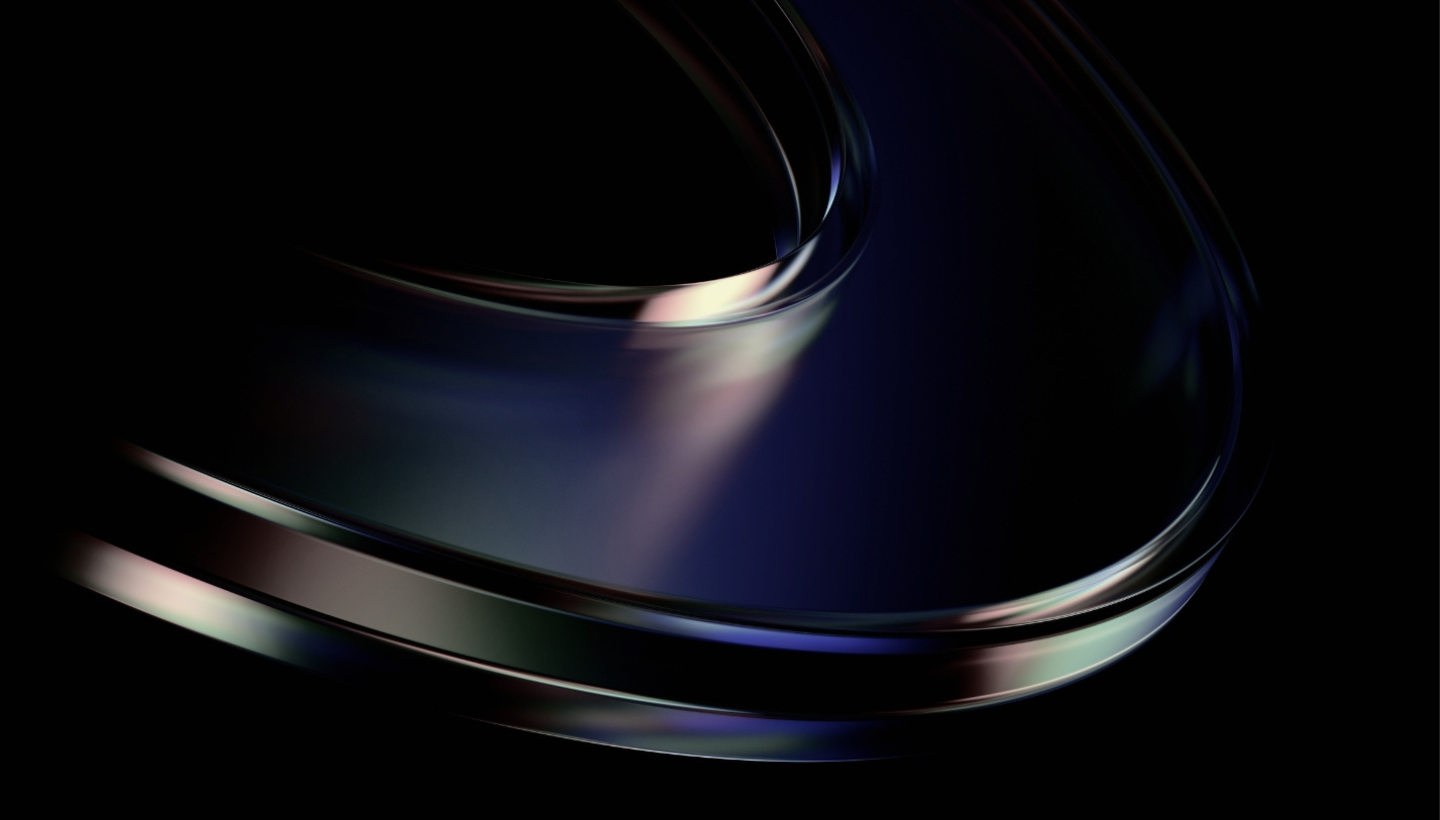
Getlink turns to AI to optimize the efficiency of its rail network

Thanks to our approach focusing on Artificial Intelligence (AI) and Generative AI (GenAI), Getlink SE, formerly Eurotunnel, the concession holder of the Channel Tunnel infrastructure until 2086, as well as operator of transport and logistics services, is working with Ekimetrics to transform, secure, and optimize its operations. Key challenges include implementing predictive maintenance and improving rolling stock reliability to help the non-quality indicator on the network drop 15% by 2026, in particular by fitting sensors on the rolling stock.
What we did
Challenge
- Improve the reliability and safety of Channel Tunnel operations and transport services thanks to AI
- Reduce maintenance costs for older rolling stock while minimizing the risk of major breakdowns
- Reduce non-quality on the network due to unplanned downtime or breakdowns
Our approach
- Bring together the data specialists and executive committee members to identify the most critical AI use cases for this project
- Move the data architecture towards "AI Readiness" and be able to support the deployment of the 12 identified GenAI use cases
- Deploy GenAI solutions to launch a game-changing predictive maintenance approach
Outcome
- Annual savings linked to predictive maintenance, estimated to exceed €1.5M
- Implementation of a predictive maintenance system for the rolling stock, which will help significantly reduce unplanned incidents and downtime
- Development and deployment of a robust, unified, and resilient AI platform
Challenge
Getlink faces increasing complexity in managing its infrastructure and rolling stock due to the challenges of resource-intensive and time-consuming maintenance as well as the need for better risk control. Rolling stock in service since the 90s, maximum safety requirements due to the strategic importance of the Channel Tunnel, and a train running every 5 minutes at peak times necessitate a fundamental review of maintenance and monitoring practices.The transition to proactive operation management implied a significant ramping-up of Getlink's AI and GenAI capabilities to foresee breakdowns before they caused major interruptions and high maintenance costs. Turning to AI was essential for continuous monitoring and efficient predictive maintenance of the aging rolling stock and complex infrastructure.
Our approach
Further to recruiting two in-house data scientists, Getlink chose us to support their transformation, as a recognized pure player and for our high level of expertise in data science, AI, and GenAI.First, we identified and prioritized AI use cases on the rolling stock. Ekimetrics and Getlink teams then defined and technically structured a robust AI architecture. It is integrated seamlessly into the existing information and operational systems. From now on, data can be collected and analyzed in a more structured, secure way, including for sophisticated projects such as those using GenAI.One of the first use cases rolled out was to reduce the phenomenon of "brake application" that can bring a train to a standstill. Its maintenance accounts for an annual cost of at least €1.5 million. The early detection of this phenomenon has proved extremely effective, thanks to the implementation of automated analysis of weak signals. Furthermore, the time saved in diagnostics for the maintainers has been devoted to a more in-depth exploration of the equipment; a further step in detecting the risk of failure.This work is currently underway on rolling stock and tunnel infrastructure and will extend to three other areas of the AI roadmap:
- Remote diagnostics to support drivers remotely in real time
- Optimization of maintenance schedules and spare parts stock levels
- The implementation of an RAG (retrieval augmented generation) trained on defined procedures and similar incident cases, to enable maintainers to get fast, accurate answers.
This roadmap currently receives funding of up to €2.5 million, broken down over the period up to 2025.
Outcome
- Increased operational efficiency: highly reliable early detection of unplanned stoppages (one of the project's major successes)
- Significant cost savings: due to drastic reduction in human effort and operating expenses linked to maintenance teams
- Improved security: increased operational security and network reliability, thanks to GenAI approaches that detect anomalies before they become critical