Thought Leadership
How to combine business approaches, advanced statistics, and technology?
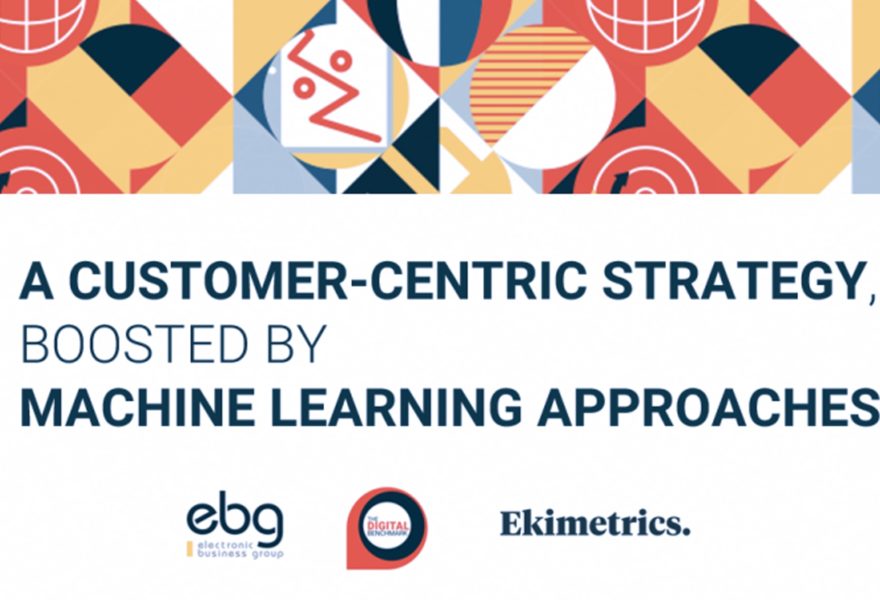
Sustain your data performance to accelerate achievement of your strategic goals
From the outset, our machine learning operations (MLOps) standards aim to industrialize each stage of the life cycle of a machine learning project, from a reproducible experimentation phase to deploying the chosen model.
Generate value from your data science initiatives by putting your models into production
Accelerate your data roadmap with our MLOps methodology
Ensure your data is precise and improve prediction quality (Monitor and maintain performance of the models that are deployed)
Enhance the productivity and the value of your business
Industrialize data science to improve business performance
Moving successfully from PoC to production: that’s the MLOps method! We are obsessed with sustaining the entire life cycle of a machine learning project to implement your use cases faster. The life cycle includes several essential processes:
Experimenting to calibrate performance
Machine learning is experimental by nature. The first phase of the MLOps cycle aims to accelerate experimentation and development of the models. How? By testing different models and features to find an approach that works and demonstrate that the model is reliable and reproducible. Results are guaranteed thanks to experience tracking. Once the machine learning process is streamlined, model training is commercialized in order to:
Operationalizing model training for increased agility
This stage consists of developing model training and prediction standards (from data preparation and transformation to model training and evaluation). Our data experts define automation principles by deploying training pipelines. The aim is to choose the best models and integrate them into the inference process.
Ongoing training makes it possible to re-train the model and react to new data, code changes or new parameters.
Once the model has been trained and validated, it is packaged, re-tested and versioned before being sent to a model registry for deployment.
Choosing the right architecture for inference
Whatever the need, be it batch or real time, we make predictions the core of your data architecture design. Here, the challenge is in verifying the reliability of the prediction results while continuing to integrate new data.
Monitoring the efficiency and effectiveness of the deployed model
After the model is deployed in its target environment, it delivers its insights for your use case.
Our experts monitor it proactively and automatically to identify any data drift that could affect the data and re-train it to ensure it remains robust and efficient over the long run.
Key skills
Thought Leadership
Thank you for your interest in Ekimetrics. Please fill out the form to ask your question.